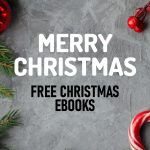
Deep Learning
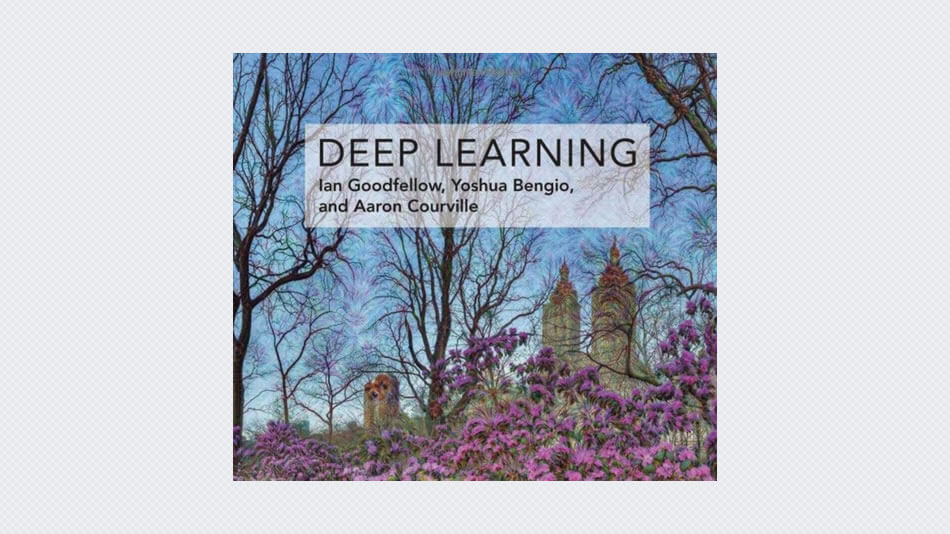
Inventors have long dreamed of creating machines that think. This desire dates back to at least the time of ancient Greece. The mythical ?gures Pygmalion,Daedalus, and Hephaestus may all be interpreted as legendary inventors, and Galatea, Talos, and Pandora may all be regarded as artificial life (Ovid and Martin,2004; Sparkes, 1996; Tandy, 1997).
When programmable computers were ?rst conceived, people wondered whether such machines might become intelligent, over a hundred years before one was built (Lovelace, 1842). Today, artificial intelligence(AI) is a thriving ?eld with many practical applications and active research topics. We look to intelligent software to automate routine labor, understand speech or images, make diagnoses in medicine and support basic scientific research.
In the early days of artificial intelligence, the ?eld rapidly tackled and solved problems that are intellectually difficult for human beings but relatively straight-forward for computers-problems that can be described by a list of formal, mathematical rules. The true challenge to artificial intelligence proved to be solving the tasks that are easy for people to perform but hard for people to describe formally-problems that we solve intuitively, that feel automatic, like recognizing spoken words or faces in images.
This book is about a solution to these more intuitive problems. This solution is to allow computers to learn from experience and understand the world in terms of a hierarchy of concepts, with each concept defined through its relation to simpler concepts. By gathering knowledge from experience, this approach avoids the need for human operators to formally specify all the knowledge that the computer needs. The hierarchy of concepts enables the computer to learn complicated concepts by building them out of simpler ones. If we draw a graph showing how these concepts are built on top of each other, the graph is deep, with many layers. For this reason,we call this approach to AI deep learning.